Supporting Wikipedia's manual review pipeline through personalized AI-assisted topic triaging.
Using topic prediction to reduce review backlog and support expert routing
📘 This post is an accessible read of the publication:
Asthana, Sumit, and Aaron Halfaker. “With few eyes, all hoaxes are deep.” Proceedings of the ACM on Human-Computer Interaction, 2.CSCW (2018): 1–18. https://doi.org/10.1145/3274283
This blog version summarizes the core motivation, data science process, and findings in a more readable, less academic format. If you’re curious about Wikipedia workflows, AI-assisted quality control, or just like cool applications of machine learning in collaborative communities — read on!
Wikipedia flips the traditional publication model: publish first, review later. But with thousands of new articles created each month, the platform faces a growing challenge — how to efficiently review submissions and ensure that only encyclopedic content survives.
This project tackles a critical bottleneck in that process: Time-Consuming Judgment Calls (TCJCs) — the nuanced reviews that require expertise to determine if an article subject is notable enough. These articles aren’t obvious spam or vandalism, but they’re not yet ready for the front page either.
The Review Crisis
Two groups primarily handle new article review:
- New Page Patrol (NPP) — reviews articles created directly in the main encyclopedia
- Articles for Creation (AfC) — handles submissions in the draft namespace
Both groups rely on volunteers, and both are overwhelmed. NPP and AfC maintain massive backlogs, and recent proposals to limit article creation by new editors only shift the burden — they don’t solve the problem. Worse, routing newcomers to hidden drafts reduces collaboration and delays quality content.
In contrast, edit review on Wikipedia is highly efficient:
- Stage 1: Bots filter out clear damage
- Stage 2: AI-assisted tools help catch borderline edits
- Stage 3: Human editors review through personalized watchlists
There’s no comparable multi-stage system for new articles — just one layer of triage by a small group of humans.

Our Solution: Predict Topics to Route Reviewers
We propose building an AI-augmented pipeline for new article review — starting with a topic prediction model to help match articles with subject-matter experts early in the review process.
Key idea
Instead of waiting for editors to manually tag drafts with topics (often inconsistently), we use Wikipedia’s folksonomic structure — WikiProjects and their broad categories — to train a model that assigns drafts to topic areas automatically.
Dataset: drafttopic
We created a large-scale open dataset of new Wikipedia drafts using the drafttopic
API and a custom WikiProjects parser. This dataset allows for:
- Multi-label classification over 43 broad WikiProject categories
- Dynamic updates as WikiProjects evolve
- Tagging of new and historical drafts at scale
Wikipedia Dump Processing & Draft Extraction
Our pipeline begins with parsing the full monthly Wikipedia XML dumps (100+ GB compressed) to extract all articles in the Draft namespace. We use a scalable SAX-style parser (mwxml
) to stream pages and filter them with:
-
page.namespace == 118
(Draft namespace) -
revision.text
present (non-empty articles) -
title
does not contain “/” (exclude user sandbox subpages)
We then enrich each article with metadata like creation timestamp, creator, and revision history.
import mwxml
for page in mwxml.Dump.from_file(open('enwiki-latest-pages-meta-history.xml.bz2')):
if page.namespace == 118 and not "/" in page.title:
for revision in page:
text = revision.text or ""
if text.strip():
save_draft_article(page.title, revision.timestamp, revision.contributor)
Data Balancing & Preparation for Modeling
The draft dataset is naturally imbalanced: some WikiProjects (e.g. Biography) dominate, while niche topics (e.g. Military History, Medicine) are underrepresented. To prepare the data:
-
We use multi-hot encoding for WikiProject tags (multi-label problem)
-
We apply undersampling of majority labels and oversampling of minority labels using a controlled hybrid approach
-
We extract features using:
-
Bag-of-Words (TF-IDF)
-
FastText embeddings averaged over article tokens
We split the dataset into stratified train/test folds while preserving label distribution.
from sklearn.model_selection import train_test_split
from sklearn.preprocessing import MultiLabelBinarizer
mlb = MultiLabelBinarizer()
y = mlb.fit_transform(draft_labels)
X_train, X_test, y_train, y_test = train_test_split(X, y, stratify=y)
Model Overview
We trained a multilabel classifier to predict topics for new Wikipedia drafts using:
- Text features: Bag-of-words and fastText word vectors
- Labels: Community-curated WikiProject categories
- Classifiers: Logistic regression, one-vs-rest models
Code snippet: Baseline model with Scikit-learn
from sklearn.linear_model import LogisticRegression
from sklearn.multiclass import OneVsRestClassifier
from sklearn.feature_extraction.text import TfidfVectorizer
# Preprocess draft text
vectorizer = TfidfVectorizer(max_features=10000)
X = vectorizer.fit_transform(draft_texts)
y = draft_labels # multi-hot encoded WikiProject tags
# Train classifier
clf = OneVsRestClassifier(LogisticRegression())
clf.fit(X, y)
📊 Evaluation Metrics
To evaluate our topic prediction model, we use standard multilabel classification metrics:
- Precision: What proportion of predicted topics are actually correct?
- Recall: What proportion of relevant topics are successfully predicted?
- F1 Score: Harmonic mean of precision and recall, providing a balanced measure.
- Coverage Error: Measures how far down the ranked list of topics we have to go to cover all true labels.
- Subset Accuracy: Percentage of examples where all predicted labels exactly match the true set (strict metric).
We report both macro and micro versions of these metrics:
- Macro averages over all labels equally, highlighting performance on rare topics.
- Micro aggregates over all instances, reflecting overall system performance.
Below is the precision-recall (PR) curve for our best-performing model across all 43 topic categories:
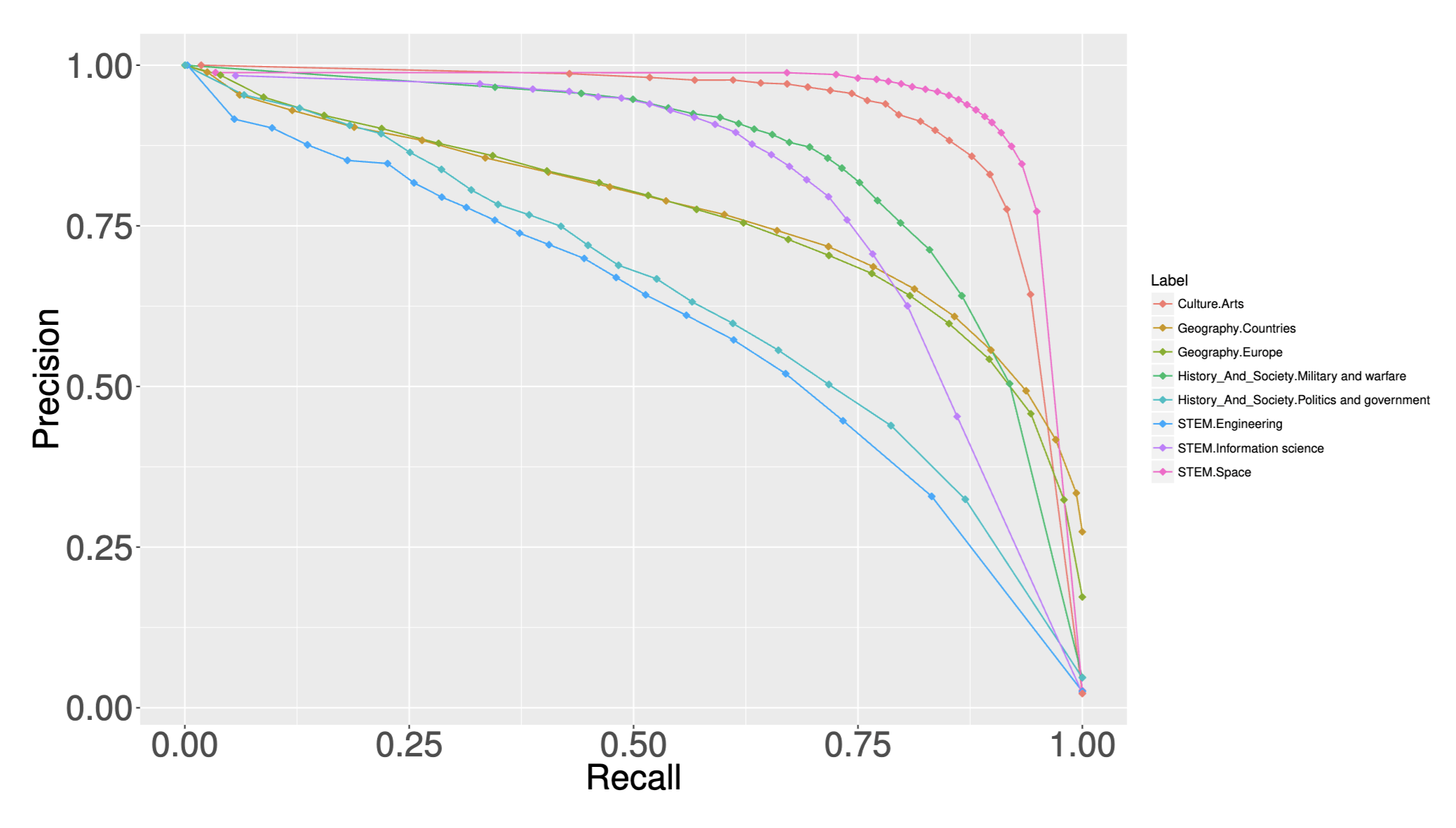
The curve shows high performance in high-confidence regions, indicating the model’s utility in supporting triage through confidence-based routing.
🔍 Insights from Topic Prediction on Drafts
Our analysis surfaced several key takeaways:
- Early drafts contain rich topical signals – Even just the opening sentences of a draft can be used to reliably predict WikiProject-level topics.
- Wikipedia’s folksonomies are machine-actionable – Despite their grassroots origins, WikiProjects form a surprisingly consistent label set that works well for ML tasks after normalization.
- Topic predictability varies by category – Technical domains (e.g., “Computing”, “Medicine”) are easier to identify, while broad domains (e.g., “Culture”, “Society”) show significant overlap and ambiguity.
- Model confidence is a useful signal – High-confidence predictions align with clearer cases; low-confidence predictions often highlight time-consuming judgment calls (TCJCs).
These insights can help reviewers better triage new drafts—by prioritizing ambiguous, low-confidence drafts that most need expert attention.
🚀 Future Directions
This work opens up several avenues for tooling and research:
- Reviewer Routing Interfaces: Develop UI tools to route drafts based on predicted topic and model confidence to the most appropriate WikiProject reviewers.
- Interactive Topic Feedback: Allow reviewers to adjust model-predicted topics, creating a human-in-the-loop system that continuously improves via feedback.
- Ontology-Aware Modeling: Incorporate the hierarchical structure of WikiProjects (e.g.,
Biography > Scientists > Biologists
) to support more granular and semantically aware predictions. - Explainable AI Integration: Highlight influential words or phrases in the draft that led to a given topic prediction to improve reviewer trust and transparency.
- Tooling for Draft Backlogs: Integrate predictions into the Page Curation Tool and AfC dashboards to offer suggested topics for new drafts at scale.
Together, these next steps can move Wikipedia’s article review from reactive backlog management to proactive, intelligent triage.