Improving AI models by labeling and learning from implicit behavior patterns
Using RNN models to detect quality issues in Wikipedia sentences
đ This post is an accessible read of the publication:
Asthana, Sumit, et al. Automatically labeling low quality content on Wikipedia by leveraging patterns in editing behaviors. Proceedings of the ACM on Human-Computer Interaction, 5.CSCW2 (2021): 1â23. https://doi.org/10.1145/3479601
This blog post breaks down the motivation, dataset, and key takeaways from our research on identifying low quality content on Wikipedia using patterns in how people edit. Itâs written for anyone interested in content moderation, collaborative platforms, or applying machine learning in real-world editorial workflows.
Wikipedia, the worldâs largest online encyclopedia, faces a significant challenge: maintaining high-quality content across its 6.5 million articles with a declining number of editors. This project demonstrates how machine learning can automate quality assessment by training recurrent neural networks (RNNs) to detect sentences requiring improvement based on semantic intent patterns in historical edits.
The Challenge
Wikipedia editors manually assess article quality using the WP1.0 Article Quality Assessment scale, ranging from basic âstubsâ to exemplary âFeatured Articles.â With article maintenance becoming a primary focus and editor numbers declining, automated quality assessment has become increasingly important.
Our Approach: Learning from Wikipedia Editorsâ Behaviors
Previous attempts at automating quality assessment have relied on crowdsourced labeling, which often produces noisy results as crowdworkers lack familiarity with Wikipediaâs specific quality policies. Our method leverages the implicit knowledge embedded in Wikipedia editorsâ actual editing behaviors.
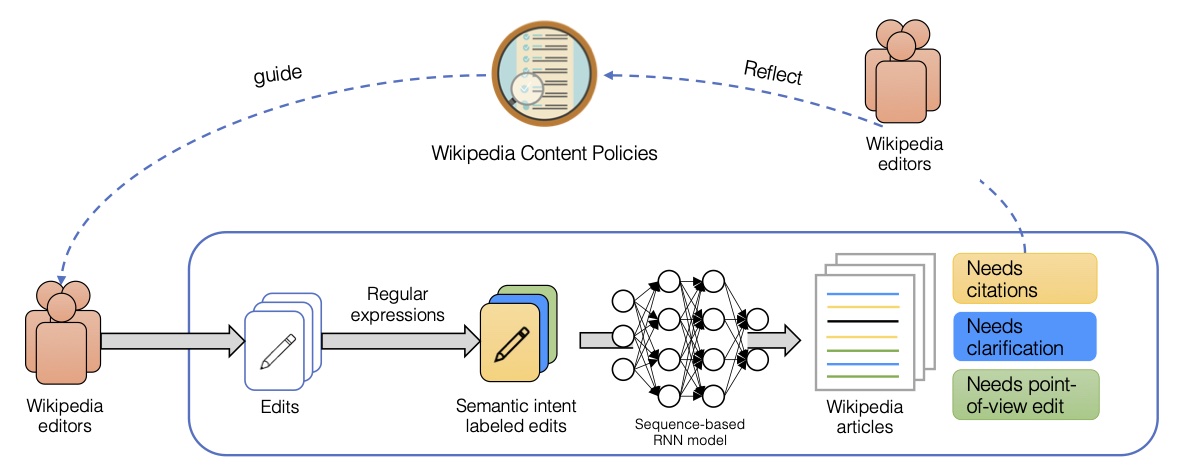
We developed syntax-based rules that capture the semantic intent behind historical edits, automatically labeling sentences that needed specific improvements:
- Citations - Adding or modifying references for verifiability
- Neutral Point of View (NPOV) - Rewriting to remove bias and maintain neutrality
- Clarifications - Specifying or explaining existing facts without adding new information
Technical Implementation
The project involved several key technical components:
1. Automatic Labeling Pipeline
We created rules to identify the semantic intent of historical Wikipedia edits by analyzing edit patterns, contextual clues, and structural changes. This allowed us to automatically generate labeled datasets for each quality category.
def extract_edit_intent(before_text, after_text):
# Identify citation edits
if contains_citation_addition(before_text, after_text):
return "CITATION_NEEDED"
# Identify neutral point of view edits
elif contains_npov_changes(before_text, after_text):
return "NPOV_ISSUE"
# Identify clarification edits
elif contains_clarification(before_text, after_text):
return "NEEDS_CLARIFICATION"
return "OTHER"
2. RNN Model Architecture
We implemented a bidirectional LSTM network with attention mechanisms to process Wikipedia sentences and predict quality issues:
class WikiQualityRNN(nn.Module):
def __init__(self, vocab_size, embedding_dim, hidden_dim, output_dim,
bidirectional=True, dropout=0.3):
super().__init__()
# Embedding layer
self.embedding = nn.Embedding(vocab_size, embedding_dim)
# Bidirectional LSTM
self.rnn = nn.LSTM(embedding_dim, hidden_dim, bidirectional=bidirectional,
batch_first=True, dropout=dropout)
# Attention mechanism
self.attention = nn.Linear(hidden_dim * 2, 1)
# Output layer
self.fc = nn.Linear(hidden_dim * 2, output_dim)
def forward(self, text, text_lengths):
embedded = self.embedding(text)
# Process through LSTM
packed_output, _ = self.rnn(embedded)
# Apply attention
attention_weights = torch.softmax(self.attention(packed_output), dim=1)
context_vector = torch.sum(attention_weights * packed_output, dim=1)
# Final prediction
return self.fc(context_vector)
3. Validation with Wikipedia Editors
To validate our approach, we conducted a user study with nine Wikipedia editors who manually labeled the improvement category of 434 historical edits. Comparing our rule-based labels with their manual assessment confirmed the effectiveness of our approach while also revealing significant ambiguity in human labeling.
Results and Impact
Our models significantly outperformed previous approaches, achieving:
- 29% improvement in F1-score for citation detection
- 22% improvement in F1-score for NPOV issue detection
These results demonstrate that learning from Wikipedia editorsâ implicit knowledge provides better signals for quality assessment than labels generated by crowdworkers who lack context.
Applications and Future Work
This research has significant implications for:
- Wikipedia maintenance - Helping prioritize editor attention to sentences most in need of improvement
- Content governance - Supporting collaborative content spaces with decentralized policies
- Knowledge quality - Improving the overall quality of the worldâs largest encyclopedia
Future work will expand this approach to additional quality dimensions and explore how these techniques could be applied to other collaborative content platforms.
Industrial Applications
The techniques developed in this research have broad applications beyond Wikipedia:
- Content quality monitoring systems for enterprise knowledge bases
- Automated fact-checking tools for news organizations
- Quality assessment for collaborative documentation systems
- Educational tools for improving writing quality and citation practices
By learning from the implicit knowledge of expert editors rather than explicit rules, our approach captures the nuanced understanding that develops through practice and community interactionâa valuable insight for any system dealing with collaborative content creation.